In the rapidly evolving landscape of education, machine learning for personalized learning has emerged as a revolutionary approach. By leveraging data-driven insights, educational institutions are now tailoring learning experiences to suit the unique needs of each student. This transformation is not only increasing student engagement but also improving outcomes at scale. In this article, we’ll explore how machine learning (ML) is powering personalized education, the benefits it offers, real-world applications, and what the future holds.
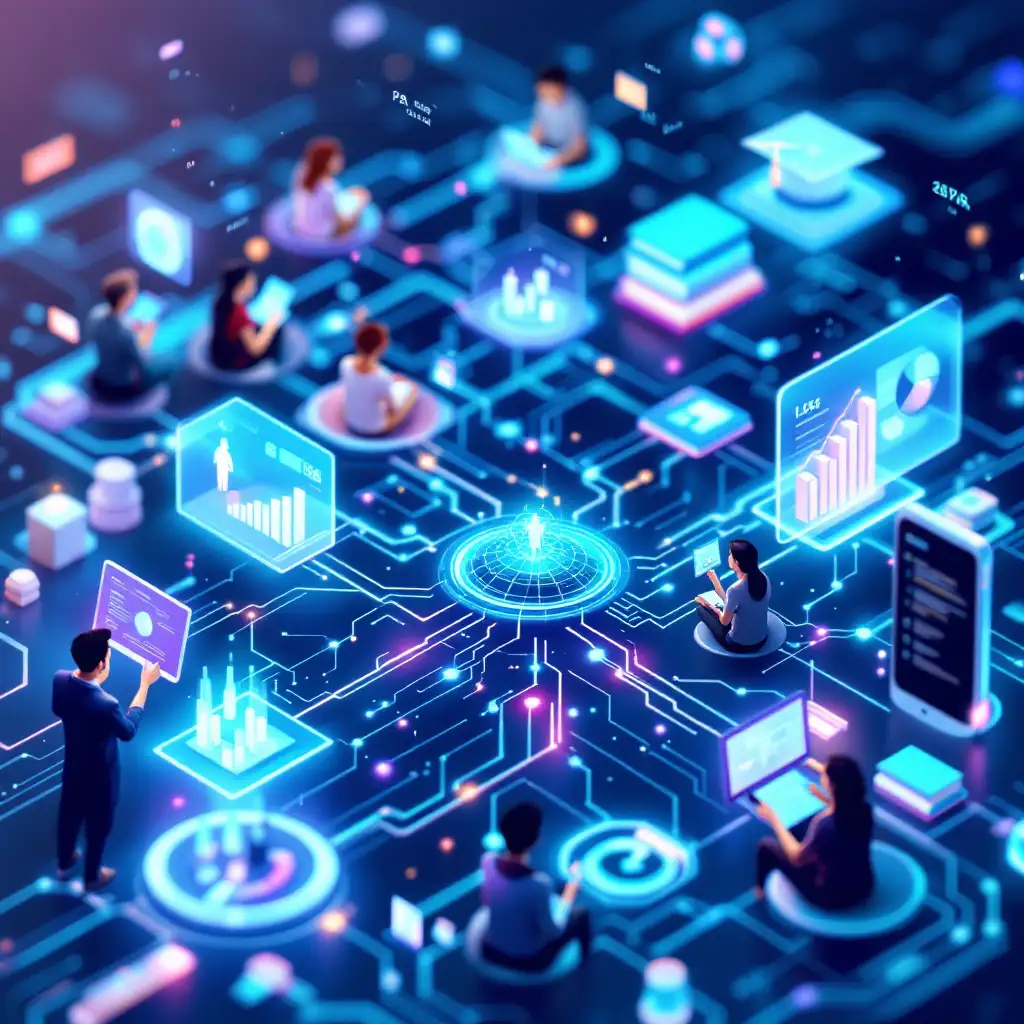
What Is Personalized Learning?
Personalized learning is an educational approach that aims to customize learning paths based on an individual student’s strengths, weaknesses, preferences, and pace. Unlike the traditional one-size-fits-all model, personalized learning:
- Adjusts content delivery methods for different learning styles
- Allows students to progress at their own pace
- Uses real-time feedback to guide learning
- Prioritizes mastery over memorization
This approach has gained popularity thanks to the rise of AI in education, particularly machine learning algorithms that can analyze vast amounts of student data to inform decisions.
The Role of Machine Learning in Personalized Education
Machine learning plays a pivotal role in making personalized learning possible. It enables adaptive learning by collecting and analyzing data points such as:
- Student responses and test results
- Time spent on lessons or questions
- Behavioral patterns during quizzes
- Preferences in content format (text, video, audio)
These insights allow educational platforms to adjust content in real-time, recommend next steps, and even predict future performance.
“With machine learning, we’re not just teaching students — we’re learning how students learn.”
— Dr. Rose Luckin, Professor of Learner-Centred Design
How Machine Learning Enables Personalization
Let’s break down how machine learning systems adapt learning experiences for individual students:
1. Data Collection and Analysis
Modern LMS (Learning Management Systems) like Canvas or Blackboard collect massive amounts of user data. ML models process this data to detect patterns, identify knowledge gaps, and predict future performance.
2. Adaptive Content Delivery
ML algorithms can dynamically present content that aligns with a student’s current understanding. For instance:
- A student struggling with fractions may get remedial videos and interactive exercises.
- A fast-learner could be given advanced problem sets to stay challenged.
3. Intelligent Feedback
Instead of waiting for human grading, students receive instant, personalized feedback powered by ML models. This promotes active learning and encourages students to revise and improve continuously.
Real-World Applications of Machine Learning in Education
Intelligent Tutoring Systems (ITS)
Platforms like Carnegie Learning and Squirrel AI provide intelligent tutoring by simulating human tutor behaviors. These systems:
- Diagnose student weaknesses
- Offer targeted practice
- Track improvement over time
Adaptive Learning Platforms
Some popular examples of adaptive platforms include:
- Knewton – Adjusts content based on student mastery level
- DreamBox – An adaptive math platform for K–8 students
- Coursera – Uses ML to recommend courses and personalize learning paths
Predictive Analytics
Educational institutions are using ML to forecast academic outcomes. For instance, early-warning systems predict which students are at risk of failing, allowing timely intervention.
External Source: World Economic Forum on AI in Education
Benefits of Machine Learning in Personalized Learning
1. Improved Learning Outcomes
By focusing on mastery, students can understand concepts thoroughly rather than just completing syllabi. This leads to:
- Higher test scores
- Better long-term retention
- Increased student confidence
2. Enhanced Engagement
ML allows students to learn through their preferred media and at a comfortable pace, reducing frustration and increasing motivation.
3. Teacher Efficiency
Teachers can use ML tools for:
- Automating grading
- Creating custom assignments
- Monitoring student progress in real time
This frees them to focus on mentorship and critical thinking development.
Challenges and Ethical Considerations
Despite the advantages, there are significant challenges to consider.
1. Data Privacy
Collecting student data requires careful handling. Institutions must adhere to regulations like:
- FERPA in the US
- GDPR in the EU
External Source: OECD on AI and Student Data
2. Algorithmic Bias
ML models may inherit biases from training data, leading to unfair treatment of certain groups. To combat this, transparent and ethically designed algorithms are crucial.
3. Accessibility and Infrastructure
Low-income schools may lack the necessary infrastructure to implement ML-based systems. This creates a digital divide in access to quality education.
Future of Personalized Learning with Machine Learning
1. Integration with Immersive Technologies
The fusion of ML with AR (Augmented Reality) and VR (Virtual Reality) can create immersive learning experiences. Imagine:
- Virtual science labs powered by ML to guide students in real-time
- History lessons in virtual museums adapting based on curiosity patterns
2. Lifelong Learning and Career Mapping
Platforms can use ML to:
- Recommend learning paths for professionals
- Suggest career options based on skills and interests
- Provide ongoing skill assessment and feedback
3. Teachers as Facilitators
In AI-enhanced classrooms, teachers will evolve into:
- Learning designers
- Emotional intelligence coaches
- Collaboration facilitators
Internal Link: Learn more about AI-powered EdTech solutions here
Conclusion
Machine learning for personalized learning isn’t just a buzzword — it’s reshaping education from the ground up. By understanding each student’s unique learning needs and adapting accordingly, we are paving the way for a smarter, fairer, and more efficient educational system.
The key lies in balancing technology and humanity — using ML to empower teachers and students while maintaining ethical responsibility and ensuring inclusive access.
Want to stay updated on the latest in educational technology?
Subscribe to our AI in Education Newsletter.
FAQs
What is the difference between personalized learning and adaptive learning?
Personalized learning is a broader strategy that may involve adaptive tools but also includes human elements like mentoring. Adaptive learning specifically uses technology to adjust content based on performance.
Are machine learning-based education tools accurate?
They can be highly accurate when built on robust data sets and continuously improved. However, human oversight is necessary to address ethical concerns and maintain fairness.
How can my school start using machine learning for personalized learning?
Start by adopting platforms like DreamBox or Knewton, ensure teacher training, and establish policies for data ethics and security.